Artificial intelligence appears on the cusp of transforming industries and changing lives at a pace we have never seen before. By analyzing vast amounts of data from sensors and other sources, AI algorithms can detect patterns and make predictions that were previously impossible. This allows us as water professionals to solve complex water problems and unlock new possibilities.
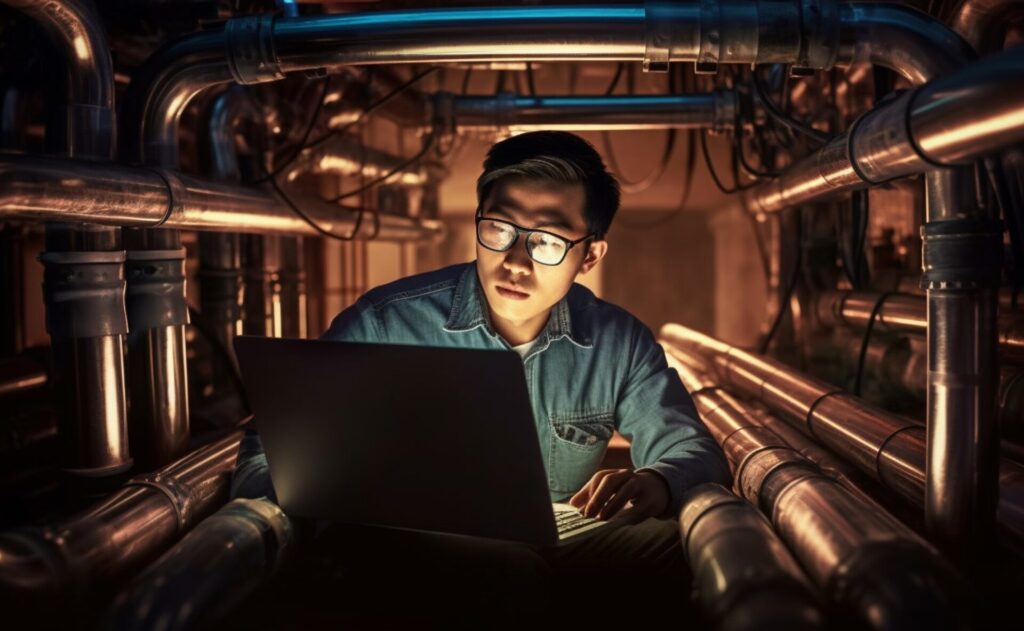
Can AI help predict sewer flows?
One example of AI in action is in predicting sewer flows. Sewer systems are critical infrastructure that transport wastewater to treatment plants, but with the impacts of climate change, aging infrastructure, and historical design practices, too much raw sewage is being released into our environment, causing harm to our planet and damaging properties.
The problem is a big one. According to a US EPA study released in 2000, of 59 Sanitary Sewer Overflows in 18 states, an estimated 1.2 billion gallons of sewerage was released. An EPA report to Congress also documented 22 billion gallons of untreated wastewater discharged into the Great Lakes Basin in 2014. It is a challenge faced by locales all over the world, most recently in the UK with Combined Sewer Overflows releasing raw sewage into rivers and the sea.
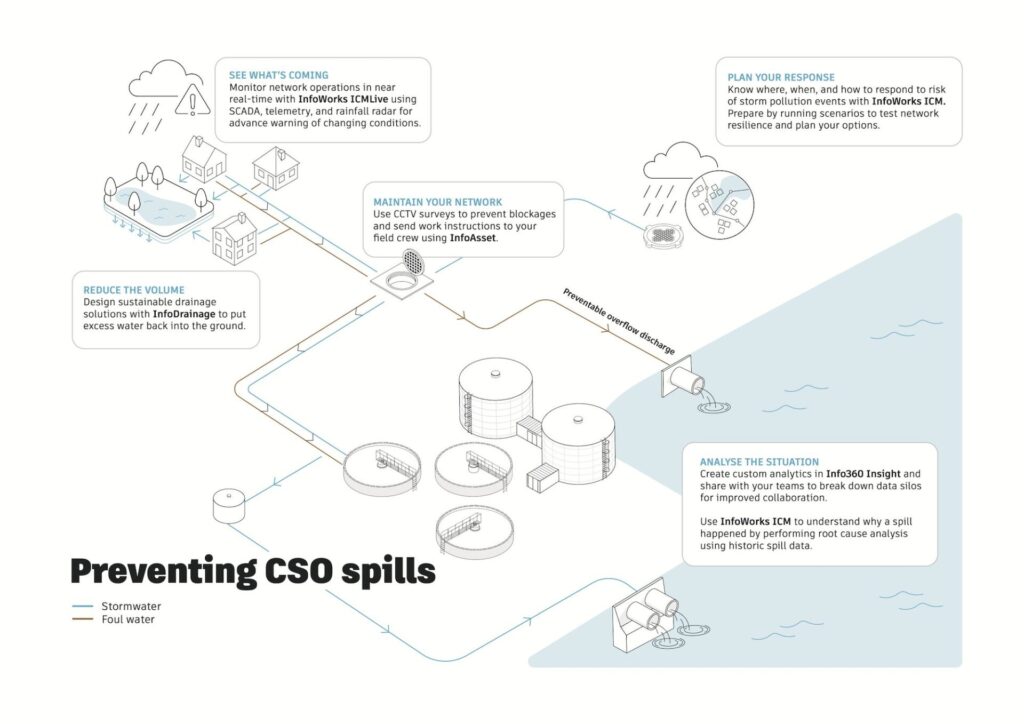
Traditionally, sewer flow predictions relied on physical models using sophisticated mathematical formulas requiring accurate input parameters. Building such models can be time-consuming and difficult, especially when input parameters are not directly measurable or reliable. A tedious and time-consuming calibration is needed to tweak the parameters to match observed flows. With AI, predicting sewer flows is more straightforward. By training an AI model with thousands of examples, the model can learn to predict the next flow value, without the need to know the exact processes.
However, building an effective AI model is not as simple as throwing data at it. It requires a deep understanding of the data, and how a human being can learn from it. The reason is quite simple. What makes modern neural network AI models so effective is that they are inspired by the human brain. The way it works is not that different from how we can finish our best friend’s sentence. Once you’ve spent a lot of time together, you learn to anticipate each other’s thoughts.
An approach to AI modeling for sanitary sewer hydrology
In our recent presentation on this topic, we found our decades experiences of calibrating sewer models played an important role in designing an effective training scheme of the AI model. By utilizing the same techniques an experienced modeler will use to calibrate a model, we were able to achieve good results.
The approach is similar to how you might learn to swim. You train on easier subtasks before combining them to tackle a complicated task. For example:
- Dry weather flows: Instead of asking the AI to learn everything at once, we start with the easiest task: extracting the dry weather flow from the flow data. That is, the flow pattern you see during the driest part of the year.
- Groundwater infiltration: Next, we move to extracting groundwater infiltration. Groundwater infiltration is controlled by the groundwater table. It is in general a slow and smooth process. You can easily see the pattern before and after extended heavy rain events. Therefore, we train the AI model with the data before and after rain events to learn these patterns.
- Learning about rain: Once our AI model knows how to predict both dry weather flow and groundwater flow, we can feed both in as inputs to train another AI model only during rain events, so it can “learn” what happens when it rains.
As you can see, compared with traditional modeling approaches, the modelers using AI models have a lot more freedom to try new training strategies that can ultimately change how the AI model understands the problem without writing any equations. Therefore, the modeler can expand the capabilities of the AI model just by training the model differently.
With great power comes great responsibility
The potential benefits of using AI in water for a task like this can be enormous. It has the potential to outperform existing solutions in forecasting and optimization problems to make our infrastructure more reliable and efficient through better planning, maintenance, and operations.
However, there are also important challenges and limitations of AI in water:
- Data quality and availability can be an issue, due to the limitation of existing technology and cost.
- Algorithm bias and interpretability need to be considered, particularly when applying AI models for extreme events not found in the training data.
- The black-box nature of neural network AI models can make it hard to anticipate a model’s behavior.
Considering these potential risks, careful implementation, transparency, and ongoing monitoring are essential to ensure that AI solutions are fair, reliable, and effective. Operational Analytics solutions like Info360 Insight and Info360 Plant can help our customers mitigate these risks and ensure a smoother adoption of AI.
The potential is vast – and moving quickly
Looking ahead, the potential for AI in water is vast, and continued innovation is needed to unlock new possibilities. AI can help us better understand our water resources, identify new solutions, and adapt to changing conditions. By working together, water managers, researchers, and AI experts can develop new tools and approaches to help us manage our water resources more sustainably.